AI, robotics, process automation: are they new movers in the food industry?
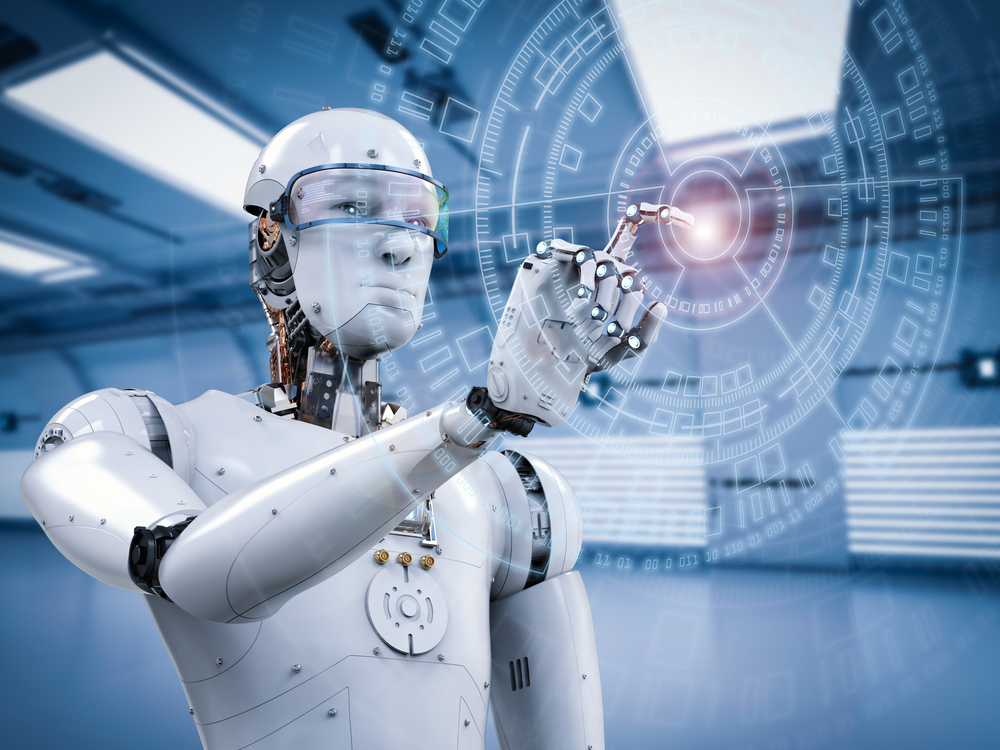
This might be a good time to review these technologies and their impact on the food industry.
Definitions
Machine Learning (ML): In machine learning, the goal is either prediction or clustering. Prediction is a process where we estimate the value of an output variable from a set of input variables. Prediction problems can be divided into two main categories: Regression problems, where the variable to predict is numerical and classification problems, where the variable to find is part of one of some number of pre-defined categories, such as “yes” or “no.” The most pronounced and common algorithms used in machine learning come in three groups: linear models, tree-based models, and neural networks. A linear model uses a formula to find the “best fit” line through the set of data points. A decision tree is a graph that uses a branching method to show each possible outcome of a decision. Neural networks refer to a biological phenomenon comprised of interconnected neurons that exchange messages with each other. This idea has now been adapted to machine learning and is called ANN (Artificial Neural Networks).
Artificial Intelligence (AI): In computer science, artificial intelligence (also called machine intelligence) refers to the imitation of human intelligence in computers that are programmed to think like humans and imitate their actions. They are computer systems able to perform tasks that typically require human intelligence, such as decision-making, reasoning, visual perception, and learn from past experiences.
Internet-of-things (IoT): It is a system of interrelated devices (such as sensors and smartphones), containing a unique identifier and the ability to transfer data over the Internet without requiring human interaction.
Deep Learning (DL): Deep learning is part of machine learning methods based on artificial neural networks. DL requires fast computers and large data sets to train large neural networks. It is has been applied to areas such as machine vision, material inspection, and bio information. Deep learning can be supervised, semi-supervised, or unsupervised.
Uses in the Food Industry
Below are several examples were AI, ML, and IoT are suggested for use:
Advance notice of food that should be recalled
One interesting use is the use of AI to predict food safety risks using social media sources such as Twitter, Facebook, and Yelp.
Online, crowd-sourced reporting tools like Yelp can provide independent, market-based incentives for restaurants and food providers to correct inferior food standards. The reason is that a report of “food poisoning” in a restaurant review can act as an independent sanction and incentive for correction. Yelp reviews were used to predict health code violations, using terms such as “sick,” “vomit,” “dirty,” or “food poisoning” to predict high risk. This system is reported to have 82% accuracy.
A site was created (iwaspoisoned.com) to crowd-source food illnesses complaints. Amazon used customers’ reviews to predict food recalls. Reportedly, this system retrieved 71-74% of unsafe food products.
Chick-fil-A tested a system to extract social media posts to identify potential food safety issues; the system had a reported accuracy of 78%.
A concern of using this AI and ML algorithms is that it could make the bias worst, rather than eliminate human bias. There is also a concern that social media users might not be a true representative of society. For example, Yelp reviewers are more likely to be white, affluent, and young.
Food manufacturing
Predictive maintenance
AI and ML can detect and predict potential safety issues before they occur and optimize time between cleaning and monitoring oil changes in commercial fryers. It allows for an automated, flexible cleaning schedule for Clean-In-Place (CIP) systems, and optimizing resources. Some systems utilize an ultrasonic sensing device and optical fluorescence imaging to assess food remains and microbial debris inside of food processing equipment.
It also can be used to optimize the cleaning schedule of bathrooms and better enforce hand washing. Employees knowing that an automated system monitors their handwashing comply better with this mandate.
Temperature control of refrigerators and incubators
The Internet-of-Things (IoT) allows the various devices to monitor, interact, and communicate with each other, track cold storage and the temperature of cooked foods, and maintain a constant temperature of food dishes. Because of their simplicity, low cost, affordability, and efficiency, time-temperature devices are widely used in the food industry. In many facilities, the temperature of incubators, refrigerators, and freezers are automatically monitored, and the data is sent electronically to users. Such a system provides unprecedented visibility and traceability, providing temperature data with time and date stamps.
In addition, the IoT sensors can also monitor humidity, pressure, and time while recording the data. In some cases, these systems can make critical decisions to ensure that the product’s safety is not compromised.
Supply Chain
AI systems can enable food products to arrive on time and avoid spoilage, helping in the accuracy of the supply ordering forecast. AI food tracking can help stores and restaurants to sell food before it spoils, through more efficiently connecting farmers with the consumers.
AI improves the traceability of food products. Its track-and-trace systems using RFID tags (tag consists of a radio transponder, a receiver, and transmitter), QR codes (Quick Response codes that can be read quickly by a cell phone), cloud data storage, and blockchain. AI algorithms where the software uses ‘Machine Learning’ to develop an understanding of factors that influence demand and automatically predict variations are being developed. The software can then permit automated ordering for supermarkets, which closely matches needs.
Artificial Neural Networks algorithms can monitor and check the process used by AI of delivery of foods and goods tracking at every step, making it safer and providing transparency to estimate the demand to avoid getting an abundance of products that end up wasted.
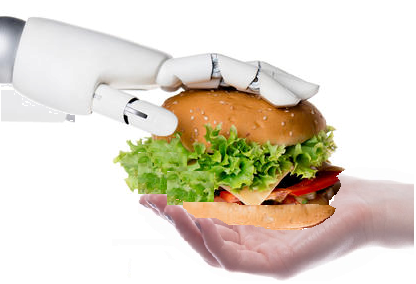
Solve workers health issues in meat plants
In meat production, we are encountering labor shortage, which is exacerbated by the COVID pandemic. Plants have closed as the virus spread through facilities. Robotic and AI can provide automated solutions and help solve the labor shortage. It can also enhance the safety of the meat.
Raw meat products tend to be irregular in shape, slimy/slippery, and delicate, making it difficult for robots to pick them up. The fast speed of the line makes the situation worse. However, recent data shows promise, especially in automating the primary packaging lines. With gripping tools, food producers can lower the risk of contamination, boost productivity, and avoid shutdowns.
Soft robotic grippers are designed to minimize the risk of spreading pathogens and are protected from the penetration of bacteria and consequent contamination. They ensure the delicate and secure handling of the meat.
Some robots consist of two mechanical hands that can take and turn over fried burger patties and put them into buns and the other ingredients needed to make burgers.
Laboratory operations
Many laboratories are plagued by closed systems that are disconnected from each other. The addition of AI systems allows effective use of lab information in a secure, open-sourced, vendor-neutral environment that can synthesize from disparate sources and systems.
AI can reduce unscheduled downtime by monitoring critical analyzer components in real-time to detect failures before they occur. The system can schedule just-in-time delivery of replacement parts, reagents, and consumables. AI could also schedule repairs or installations to be completed most conveniently and effectively. The software can also automate sample management and optimize workflow.
Sorting food
There are AI-based applications to recognize which potato should become potato chips and which are better to use for French fries. Vegetables of an inappropriate color will also be sorted out by the same system, decreasing the chance that buyers discard them. The system has sensors and a camera that recognizes material based on color, biological characteristics, and shape (length, width, and diameter).
Bottom line
The new era of food safety and the numerous publications about the food industry AI and ML in this new era generate much excitement on the potential of these technologies to reshape the industry. However, in many cases, AI went from “boom to busts,” with many promises that fell short.
There are some tasks that machines perform today, such as cow milking, picking fruits, using IoT for refrigerators, and storage. and there are predictions of compound annual growth rate of 28-50% in the next five years. However, an AI system for the food industry is not an “off the shelf” product.
While progress in the last few years has been significant, we are only at the beginning of delivering solutions with AI. The most significant advantage of AI applications in the food industry is that there would be fewer human errors, and the automated process would result in a higher quality of the product.